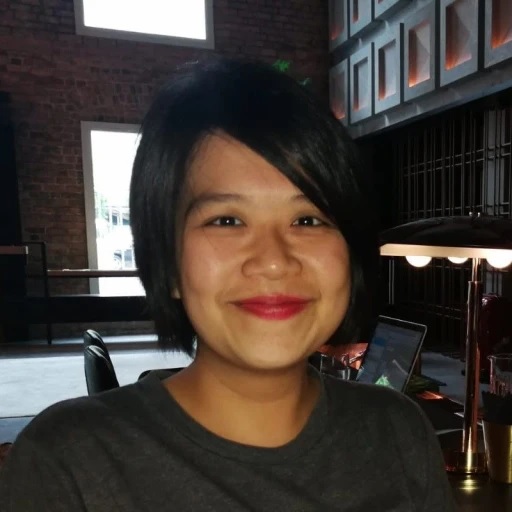
Hazel Tang A science writer with data background and an interest in the current affair, culture, and arts; a no-med from an (almost) all-med family. Follow on Twitter.
The 105th Radiological Society of North America (RSNA) Scientific Assembly and Annual Meeting took place in Chicago last December. Three prominent radiologists who attended the event share their thoughts on how radiology might look in the year 2050.
What was your key takeaway from RSNA 2019?
Dr. Shah: I realized AI is still a big topic because there was an entire exhibit hall dedicated to AI companies! This year, I also witnessed the emergence of many new Asian companies, showcasing many large and advanced setups, which shows how crowded the marketplace is right now. I spent a lot of time talking to vendors and it seems most of them are much closer than they were last year in understanding the needs of radiologists with respect to workflow and integrating products into existing RIS/PACS systems. I believe understanding the priorities of radiologists is going to determine whether some of these companies will still exist in the near future.
There is an ongoing debate about who is going to pay for these new technologies. At the moment, radiologists are unsure who is going to pay for AI – is it private practice radiology groups, hospitals, academic medical centers or insurance companies — no one knows and this is creating some concerns.
Dr. Lungren: I think the biggest news in AI at the RSNA was the 2019 ASNR (American Society of Neuroradiology) RSNA Brain CT (Computed Tomography) Hemorrhage AI Challenge. The AI theatre space was filled to capacity in anticipation of this event. Five of the top ten winners were in attendance and each gave a brief overview of how they addressed the problem of identifying and categorizing the intracranial hemorrhages. All of the winners showed tremendous creativity RSNA 2050 and innovation in their approach to the problem.
While all winners were experienced data scientists, they came from varied backgrounds and, for most, this was their first time applying their talents toward a medical application. One of the winners came from a background analyzing baseball statistics!
Altogether, the competition received 22,213 submissions from 1,345 teams made up of 1,787 individual competitors from 75 countries. The top solutions had performances better than the currently available commercial solutions and all competitors have made their models/ code available as open source.
In addition to the open source models, the 25,000 CT training dataset, donated from four hospitals in three countries labelled by expert neuro-radiologists from ASNR, now stands as one of the largest and most valuable in the world for research and educational use. The continued trend toward open source medical imaging datasets (and challenges) like this one will continue to drive innovation and engagement of the world’s best computer scientists on medical imaging solutions.
Dr. Kottler: I saw a few new AI trends at RSNA this year. First, all of the AI vendors have aligned with the human-cybernetic collaboration model and are no longer talking about replacing radiologists. Vendors were also selling improved efficiency as this may prove to be a more viable business case given the global radiologist deficit. Several new and returning AI vendors were starting to promote the zero footprint viewers and cloud based worklists they created to optimize their AI workflow. I do think AI will be the next wave of improved radiologist efficiency. The question is, will these new vendors disrupt the legacy systems?
Finally, there was a new trend in radiology hardware, specifically portable, CT and MRI systems that can be deployed quickly at a fraction of the cost of a full-scale system. While there were more AI vendors at RSNA than any year in the past, I suspect we will soon see a significant market consolidation. We have already started descending from ‘the peak of inflated expectations’ (Gartner Hype Cycle), and that trend will continue as compensated clinical adoption of AI is limited.
Outside of AI, there was a great session hosted by the American Association of Women in Radiology (AAWR) about family medical leave in academic and private practice radiology. A recording of that discussion and the associated resources should be available through the RSNA virtual meeting.
How has AI changed radiology and what are some of the biggest challenges facing radiologists right now?
Dr. Kottler: I wish AI was changing radiology more quickly than it currently is. There are hundreds of AI vendors in radiology alone, doubling every year, but unfortunately the amount of AI that’s being used clinically is pretty limited. One of the biggest barriers to adoption is that there isn’t an accepted business model for AI – who is going to pay for it? So I don’t think AI has changed radiology as much as it has the ability to. I am a huge proponent of AI and I look forward to the human- cybernetic collaboration I believe will transform everything we do in the future, but radiologists need to understand how each model works, and the limitations of those models. One such limitation is the bias implicit in AI models caused by the bias implicit in the underlying datasets. An important example is something called Data Set Drift. It is easy to assume the published accuracy of an algorithm is generalizable, when in fact it is not. If we teach an algorithm using one set of data and get good results, we have found that those results are not reproducible when the data set is different i.e if your data is different from the training data. Differences in data come in many forms from patient demographics, ethnicity, disease prevalence, imaging equipment, protocols, imaging quality etc.
Overfitting is another interesting topic. When we overfit an algorithm to our own population and are only applying it to that population, it can actually be a good thing, as it makes a more precise prediction based on optimization to that single data set. However, overfitted models are even less tolerant to differences in data and less generalizable.
Dr. Shah: I think in general, radiologists are excited about AI although some of the early thoughts were, “Oh my gosh, this is something that could replace me!” but that seems to have passed. Presently, most radiologists are really looking for an AI tool which can help them to be more efficient. Given that, I feel the state of radiology has not greatly changed for the past 20 years, since the advance of PAC and voice recognition technology. The only thing that has changed is that radiologists are getting busier in the US and they are required to review more images faster. This pressured working environment is causing burnout and I hope AI can actually alleviate a large number of problems we face in our workflow.
Dr. Lungren: Some of the biggest changes to radiology still seem to remain in the realm of what is possible. Vendors and many research presentations on the whole, lacked data to describe overcoming the challenges around sensible AI workflow integration considerations, including monitoring for bias, quality control, measuring and reporting clinical outcomes, and what impact AI ‘assist’ or ‘augment’ truly has on diagnostic performance.
These questions are essential and the answers so far are not readily available. The lack of transparent, well designed prospective integration trials or data is disappointing because it’s the way that the medical community evaluates new technologies and drugs before applying them to care of patients.
For me, the biggest challenge was outlined in the several panels convened this year around global health and radiology AI. As these technologies move toward democratizing radiology diagnosis, and the conversation has moved to assisting radiologists, it was very clear that there are simply no radiologists to assist at all in most of the world. This adds an additional burden to the application of AI, and requires a paradigm shift away from assisting radiologists and, instead, assisting non-radiologist clinicians to perform that task instead.
This might include using these technologies to enable an internal medicine doctor or a nurse practitioner to interpret an imaging examination without radiologist consultation in places where there are no immediately available radiologists.
As AI becomes more prominent, how do you see radiology in the year 2050?
Dr. Lungren: I recently read that 30 years ago there were less than 800 thousand mobile devices in the world while today there are more than five billion! Thinking back to radiology technology 30 years ago when we practiced with plain films and employed an entire profession around transcription, we could never have imagined the kinds of technologies we use daily now. Judging by the pace of technologic innovation that only continues to accelerate, it seems pretty clear that if there are radiologists practicing in 30 years from now they would be practically unrecognizable to us today.
As a technology optimist, I believe one of the biggest drivers of the future changing landscape will be
the expanding global access to powerful computing and connectivity which will further democratize medical knowledge and expertise. This will lead to a future where all clinicians (not just radiologists and cardiologists) will incorporate expert-level knowledge of medical imaging into their daily practice in a dissemination of imaging diagnostic expertise by technology – eliminating the need for interpretation and delivery within the domain of an entirely separate specialty as it is practiced now. We shouldn’t be intimidated by this but empowered. There is already a great opportunity for radiologists to steer this future state and ensure that the knowledge we have as a specialty can be delivered by the coming advances in technology and connectivity to drive more breakthroughs, creating new skills, and deliver on the potential for better healthcare for the whole world.
Dr. Kottler: I just gave a lecture to medical students and told them radiology is a fantastic career now and I think in the year 2050, it’s going to be even better. I recently showed students a picture of Tom Cruise from Minority Report, where he is standing in front of a giant computer screen, moving data around with his hand, and used it as an analogy for what I think radiologists are going to be doing in 2050. While we may not be using that specific technology I see radiologists of the future functioning as information experts, contextualizing all of the information from different AI systems and using that to consult and communicate with our referring clinicians and patients.
I see AI helping us ‘go back to the future of radiology’. In the past we spent much of our day consulting with our referring clinicians. With the advent of PACS and the increase in reading volume, much of this robust patient centric communication has disappeared. By 2050, I see AI helping us get back to that kind of collaborative patient care with radiologists again taking the role of the physician’s physician.
Dr. Shah: I think radiology is going to change in a couple of ways in 30 years from now. AI will be something ubiquitous by then. It will be incorporated in all facets of imaging technology. These technologies will assist in identifying the pathology behind the most difficult and unusual cases while humans will continue to exercise intelligence and creativity to make better diagnoses for patients.
Do you think the present generation of radiologists are prepared for that future?
Dr. Kottler: Probably not. I think there are several ways we are not preparing present young professionals for the future. Radiology touches almost every patient in the hospital so I think radiology should be a required early rotation in medical school. We also need to make sure the present generation understands the basics and can speak the language of AI and data. Radiologists don’t need to be trained on the specifics of machine learning algorithms or the statists behind each algorithm, but need to understand generally how they work so they can determine how they should be optimally used and understand the potential biases they introduce. The radiologists of the future will need to provide advice based on the knowledge generated from these systems. We also need radiologists to translate clinical use cases to AI vendors to help ensure new models are built that drive value for our patients and healthcare system. All of these require basic AI training.
Dr. Shah: I think they may not be prepared but it will come. I believe there is still time for us to catch up as the market is not matured yet; we still have not had products that are well integrated into the current radiology environment. So, once that occurs, we will be able to start seeing radiologists thinking about the more distant future.
What can be done better to prepare us for that future in radiology?
Dr. Shah: I think one of the things we really need to improve is the quality of diagnostic accuracy. We radiologists are human, so we are bound to make mistakes. The only radiologists who don’t make mistakes are the ones who are not reading cases. So, I believe AI is a great way to check and double- check radiologists’ work. It can be an incredible tool without the stigma of current archaic peer review systems which do not focus on learning.
Dr. Lungren: I think it’s important for the future radiologists to be familiar with a lot of the concepts that regrettably aren’t being taught now. We are taught sciences, how MRI works, but we are not taught how an AI system works, what is data science and bias and so on.
All these, in my opinion, will become incredibly important, probably in the next two years or even less.
While radiologists can’t build MRI machines, we do know how the fundamental concept works. The same applies for AI. We are not going to know how to make our own model, but we have to understand what the principles were that went into that model and how it was developed so that we can troubleshoot it and make sure it’s safe to be used for our patients.
Dr. Samir S. Shah. Daytime Pod Practice President, MATRIX Teleradiology (Division of Radiology Partners).
Dr. Matthew Lungren. Director of the Stanford AIMI Center; Assistant Professor of Radiology (Pediatric Radiology), Stanford University Medical Center.
Dr. Nina Kottler. Vice-President of Clinical Operations, Radiology Partners.